The healthcare industry is experiencing unprecedented data generation and collection in the present healthcare landscape. The global big data healthcare market, valued at $67 billion in 2023, is expected to grow up to $105.73 billion by 2031, showing explosive growth and the growing importance of data analytics in healthcare. This impressive growth of healthcare data and analytics is due to technological progress and the increasing need for improved health administration and optimization of patient outcomes. This blog will be your guide in understanding data analytics and how it is an important element in healthcare.
What Is Data Analytics in Healthcare?
Healthcare data analytics is that transformative force in modern medicine, which basically changed the way healthcare providers collect, process, and use patient information to better deliver care. Healthcare data and analytics generally mean the systematic analysis of raw healthcare data—including patient histories, bloodwork results, & genetic information—to uncover meaningful patterns and insights that guide evidence-based decision-making!
The transformation that data analytics brings to healthcare is revolutionary and extensive. Today, healthcare providers make use of sophisticated technologies such as machine learning and data visualization in the promotion of medical practices and efficient usage of resources. Healthcare data and analytics brought about great changes in patient care quality, management of clinical data, and higher accuracy in diagnostics, coupled with business operations. Data business intelligence has transformed patient care, offering medical professionals a complex capacity to make decisions based on data that has been extensively analyzed.
With big data analytics and healthcare, unprecedented improvement in the entire healthcare ecosystem has come along. Industry research indicates that data analysis in healthcare from big data can save the US about $300 billion annually. This is about 8% of national healthcare expenditures in a year. Such a vast impact is seen through improved diagnostics, less fraud, and better outcomes for patients.
Moreover, given the fact that more than 95% of US hospitals have recently started implementing EHR systems and over 60% have adopted IoT devices, the availability of healthcare data is unprecedented.
The Foundation of Healthcare Data And Analytics
The foundation of healthcare data and analytics is based on the different kinds of data types that comprise the basis of contemporary healthcare decisions. The huge amounts of information are collected and analyzed from various healthcare data analytics platforms by healthcare organizations, which leads to deep insights into the care provided to patients, operational efficiency, and even financial performance.
Clinical Data
The most important part of healthcare analytics is clinical data, including EHR, CPOE, medical images, lab tests, and vital signs. It will encompass all data on patient histories, blood tests, genetic factors, and other data provided by the clinical support system, thus allowing an understanding of the general state of patient health and outcomes from their treatment. All this information is processed by healthcare data analytics software for better clinical decision-making and improvement of care quality.
Financial Information
Healthcare Financial Analytics would include claims information, billing data, and all interactions with insurance. In healthcare data analysis, this plays a crucial role in which healthcare organizations can optimize the revenue cycle, manage and reduce costs, and enhance their financial performance. Claims data includes billing interactions between an insured patient with the health care provider, representing information regarding diagnosis, services performed, date of the service, and the respective cost for the service.
Operational Information
Operational analytics thrust areas for hospitals include management, resource allocation, and workflow optimization, including staffing schedules, equipment utilization, supply chain management, and patient flow data. Such information is processed in the healthcare data analytics platform so that operational efficiency and waiting times are improved, along with resources being used in the best ways possible. Advanced healthcare data analytics platforms assist healthcare administrators in identifying bottlenecks, streamlining processes and improving overall organizational performance.
Patient Behavior and Sentiment Data
This includes data from myriad sources: social media posts, smartphone health applications, patient satisfaction surveys, even behavioral health information. These data analytics yield precious insights into what patients want, what they are willing to engage in, and whether or not they stick to the recommended treatment. This allows big data analytics to personalize patient care and make better, more effective approaches toward engagement and overall delivery of health care.
It is the integration of such diversified data types that has brought sophistication to healthcare data analytics platforms in transforming patient care and management. More than ever, the healthcare sector has become a place of decisions through data, outcomes of improving patient care, and competition at an evolving level.
Key Elements of Healthcare Analytics
Healthcare data analytics platforms rely on four fundamental elements that work together to transform raw healthcare data into actionable insights. These make up the core of contemporary healthcare data and analytics systems, allowing organizations to use data correctly to benefit patients and increase operational efficiency.
Data Collection
The foundation begins with robust data collection methods aggregating information from multiple sources: EHRs, medical imaging, laboratory tests, and much more coming from the patients themselves. Healthcare data analytics software ensures that such gathered data is comprehensive, accurate, and compliant with privacy regulations like HIPAA.
Data Storage Systems
Healthcare organizations use a range of storage solutions to manage enormous repositories of data. Examples of these include on-premises, cloud-based, and hybrids that combine both methods. The choice of storage option would depend on data sensitivity, accessibility requirements, and the need for significant processing power for complex analytics computations.
Analysis Tools and Techniques
Analytically, contemporary health analytics uses three paradigms:
Descriptive analytics analyzes past data to show past trends.
Diagnostic analytics digs deeper into the root cause of healthcare outcomes
Predictive analytics predicts future trends and patient outcomes.
Data Visualization and Reporting
The last element involves taking complicated healthcare data and representing it in simple and easy-to-understand visual forms. Healthcare data analytics present the information effectively through using dashboards, interactive maps, and custom reports. Visualization tools help clinicians monitor patient results, the effectiveness of any treatment given, and relevant trends in healthcare. To that effect, user needs therefore have to be taken care of with constant feedback collection before visualization tools can be appropriately utilized in health.
These four elements enable the power of data analytics for better quality patient care, optimal operational efficiency, and evidence-based decision-making across their operations.
Benefits of Data Analytics in Healthcare
Better Patient Care
Predictive Analytics About Patient Care
One of the core Benefits of Data Analytics in healthcare is that it allows doctors to wade through large amounts of clinical data to determine responses by patients going through certain treatment protocols. These systems help doctors compare and evaluate various approaches, thus providing a diagnosis and, hence, a proper decision regarding the treatment protocol.
Customized Plans
Analyzing patient histories, medical records, and responses to treatment creates a patient-based strategy for data analytics in healthcare. It can process the data that will help make proper clinical decisions and better overall quality care.
Detection of Disease at Early Stages
One of the significant benefits of data analytics in healthcare is the ability to identify at risk patients and even ailments at their potential early stages. This approach allows for timely intervention in all the preventive measures, which has significantly improved patient outcomes.
Operational Efficiency
Resource Allocation
The analysis of hospital admission patterns and patient flow data allows healthcare data analytics platforms to optimize resource utilization. In this regard, beds in the facility can be managed efficiently by improving room scheduling, operating rooms, and the proper distribution of medical tools.
Staffing Scheduling
The benefits of data analytics in healthcare include improved staff scheduling through predictive modeling of patient demand. Trends and patterns enable healthcare facilities to improve optimal coverage for staff and reduce overtime costs.
Supply Chain Management
Healthcare big data analytics improves inventory management as well as supply chain efficiency by tracking usage patterns and predicting future needs. This would reduce the likelihood of stockouts but with optimum costs on overstocked inventory.
Financial Performance
Revenue Cycle Optimization
The benefits of data analytics in healthcare are extended even into financial operations where claims are being submitted in an easy way, increasing billing accuracy. Through identifying inefficiency in any area, a healthcare provider, with the help of data, may work to improve the payment process.
Fraud Detection
Advanced algorithms in healthcare data analytics software examine the claims data of any healthcare organization in real-time in order to find suspicious patterns and possibly fraudulent activities. They prevent loss of funds for healthcare organizations and ensure regulatory compliance as well.
Risk Management
Data analytics in healthcare is very significant to undertake proper risk assessment and management. It helps health organizations understand the potential risks earlier and adopt appropriate mitigations that ensure both financial stability and safety of patients by analyzing data regarding patients and operations.
Data analytics thus becomes an indispensable tool in modern health care. This technology changes how organizations deliver care, operate, and maintain financial health. The effect of data analytics is projected to increasingly grow as healthcare organizations seek better patient outcomes but maintain operational efficiency as well as financial sustainability.
Healthcare Data Analytics Platforms and Software
Popular Healthcare Data Analytics Platforms
Enterprise-level Solutions
Healthcare data analytics platforms such as Arcadia Analytics and Health Catalyst are enterprise solutions that provide a variety of data sources for healthcare providers, including EHR systems, claims data, and pharmacy records. These enterprise solutions deliver sophisticated analytics capabilities in both clinical and operational decision-making so that healthcare providers can achieve the best care for their patients and the best financial outcomes.
Specialized Healthcare Data Analytics Platforms
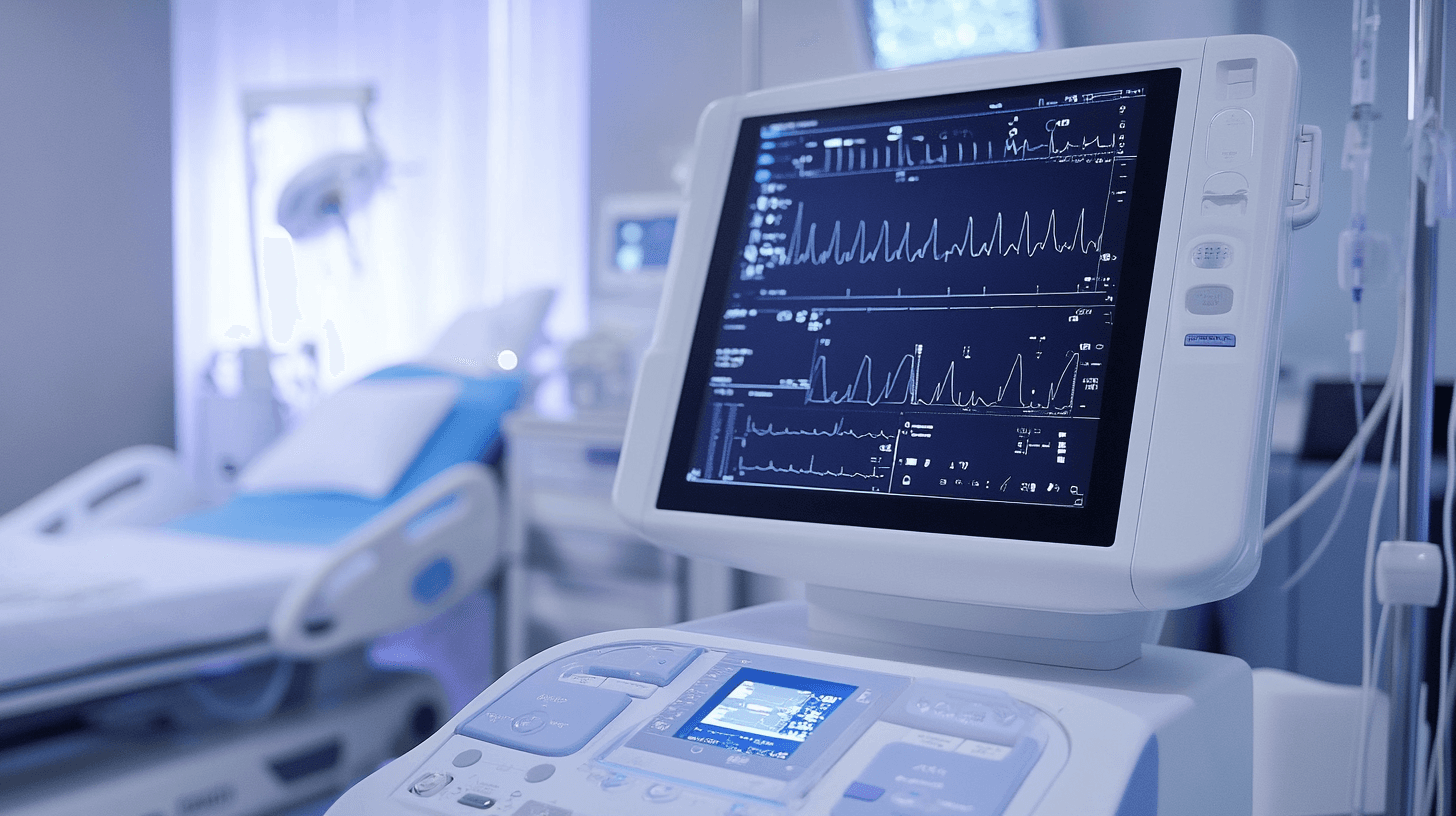
Specialized healthcare data analytics software like CareGauge and Clarify Health are specialized in particular aspects of healthcare delivery. These solutions provide clinical decision support, care utilization insights, and benchmarking. They stand out with their precise solution for the most precise problems in healthcare population health management to value-based care initiatives.
Integration Capabilities
Modern healthcare data analytics platforms have strong integration capabilities that easily connect with existing healthcare systems. They are integrated with the EHR system, practice management software, and billing systems, thus creating an integrated data ecosystem. The flow of data is smooth, and real-time analytics allow for the making of decisions at a moment's notice.
Security Features
The healthcare data analytics software gives very strong security measures that protect sensitive patient information. Encryption, access controls, and compliance with all healthcare regulations, including HIPAA, are part of the offering. Security features are woven into the platform from data gathering to analysis and reporting.
Implementation Considerations
Infrastructure Requirements
They should understand their technical infrastructure requirements in implementing analytics solutions. Thus, they have to measure the server capacity, networking capabilities, and storage demands. On the other hand, cloud-based solutions are much more flexible with much less overhead compared to on-premise solutions.
Training Requirements
Implementation requires comprehensive training of healthcare staff. This training includes technical training on the utilization of the platform and analytical skills to interpret data. Often, healthcare data analytics platforms provide ready-made training resources and supporting systems.
Cost Elements
Implementation costs will comprise software licensing, setting up infrastructure, training, and maintenance. Organizations have to consider both the cost of investment and the running costs in choosing healthcare analytics solutions.
Scalability Capabilities
Healthcare data analytics platforms need to scale with the needs of the organization. Scalable solutions enable the growth of data volumes, additional users, and new analytical capabilities. Cloud-based platforms are usually more flexible in scaling to support growing healthcare organizations.
Big Data In Health Care
The Role of Big Data
Volume of Health Data
Healthcare organizations provide various services for which patients undergo electronic health records and are involved in clinical trials by medical imaging and monitored by many devices. The processing of various information coming from different sources such as apps, portals, websites, and EHRs provides a perfect ecosystem of patient data.
Data Velocity
This trend will definitely see the speed of creating and processing healthcare data increasingly rise. Healthcare data analytics software must handle the streams from real-time data created in medical devices, patient monitoring systems, and clinical documentation in support of quick decision-making.
Variety of Data Sources
Healthcare data comes in diverse forms, including structured clinical records, unstructured physician notes, medical imaging, genomic sequences, and patient-generated data. These different types of information healthcare systems process should give healthcare providers meaningful insights.
Value Creation
Healthcare big data analytics value: improved patient outcomes, operating efficiency, and cost reduction. Healthcare organizations use these insights to create individualized treatment plans, preventive care strategies, and resource optimization.
Technologies and Infrastructure
Cloud Computing
Healthcare data analytics platforms built on the cloud provide scalable solutions to store and process enormous amounts of medical data. These systems enable safe access to clinical information, which is consistent with health regulations.
Machine Learning
Machine learning algorithms analyze large, complex datasets in healthcare to identify trends in the progression of disease, responses to treatment, and outcomes in patients. This technology increases diagnostic accuracy and allows for predictive analytics, which provides for early intervention.
Artificial Intelligence
AI in healthcare data analytics improves clinical decision support, automates routine tasks, and improves the accuracy of diagnosis. Healthcare data analytics software, based on AI, enables healthcare providers to better analyze patient data for proper treatment decisions.
Internet of Things in Healthcare
The Internet of Things device captures real-time patient information using wearables and other medical monitoring equipment. This equipment is integrated with the health care data analytics solution that provides continuous monitoring of the patient and early detection of any health-related issues.
Real-World Applications
Clinical Applications
Predicting Diseases
Advanced algorithms allow healthcare data analytics platforms in health care to identify early warning signs of diseases by pattern recognition in patient data. Various health indicators, medical history, and genetic information are used by health providers to predict potential health issues early and thereby avoid them at critical times.
Optimization of Treatment
Data analysis in healthcare allows doctors to develop individualized treatment plans based on patient-specific data. Healthcare data analytics software analyzes treatment responses, the effectiveness of medication, and patient outcomes while establishing which therapeutic approach best applies to any given case.
Drug Development
Accelerate the drug development process using big data analytics in healthcare by analyzing patient data and thereby identifying potential drug targets, as well as predicting responses to treatment. Healthcare data and analytics platforms help pharmaceutical companies optimize research and development processes, leading to better medications and shorter development times.
Clinical trial management
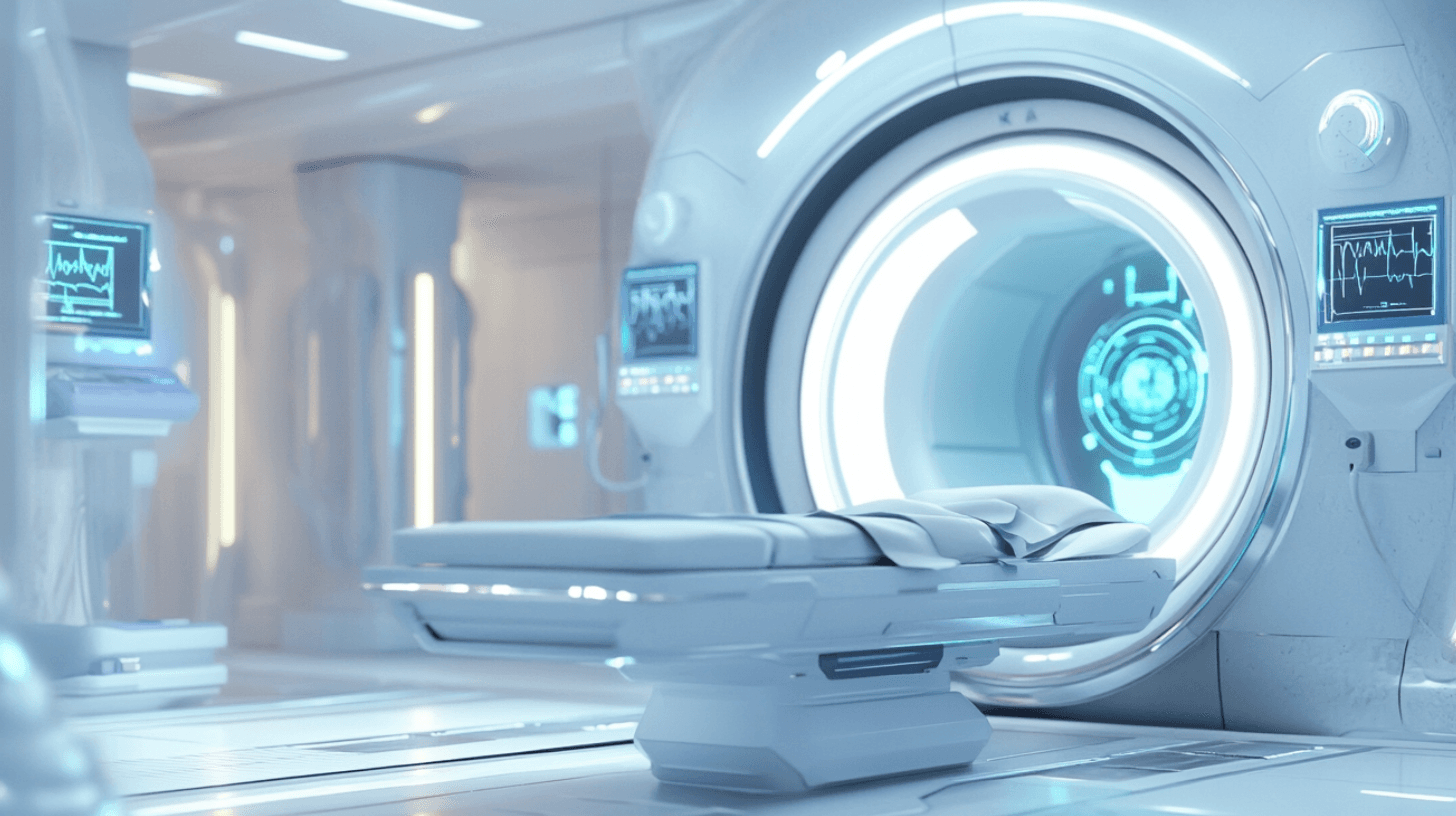
The use of data analytics in healthcare has transformed clinical trial management through the improvement of patient recruitment, protocol optimization, & real-time monitoring. Healthcare data analytics platforms help researchers identify the right candidates for a trial and track the progress of a trial much better.
Administrative Applications
Workflow Optimization
Healthcare data analytics platforms streamline the operational processes by looking into the patient flow, the trends of usage of resources, and the scheduling of personnel. This would result in minimal waiting time and ideal utilization of healthcare facilities.
Quality Metrics
Data analytics in health care allows constant follow-up of quality measures such that health care organizations have room for better care standards. Healthcare providers make use of such insights and address areas to be improved for evidence-based best practices.
Compliance Monitoring
Healthcare data analytics software is utilized by organizations to maintain compliance on many operational aspects. It tracks different aspects and automatically flags potential compliance issues with suggested correction measures.
Patient Experience Management
Data analytics is applied in healthcare as it also helps in managing better patient satisfaction through better delivery of services. Analyzing feedback received from patients, waiting times, and treatment outcomes of a patient will help organizations achieve higher levels of engagement for the patients.
Through these applications, healthcare organizations can leverage data analytics to improve both clinical outcomes and operational efficiency. This is because the significance of data analytics in healthcare is on the rise, as organizations look for more customized and effective care while maximizing their resources and staying compliant with the regulations.
Challenges & Solutions
Data Management Issues
Data Privacy and Security
Healthcare data analytics platforms should ensure confidentiality, integrity, and access to the sensitive medical information they possess. Health organizations face relentless cybersecurity threats. Therefore, robust security measures will be needed for the protection of patient information when collecting, storing, and analyzing.
Data Quality and Standardization
Healthcare data analytics software has to deal with unstructured and fragmented data from multiple sources. Due to the lack of standardization among electronic health records and variations in data formats, the job of maintaining data quality is really cumbersome. Most healthcare facilities face issues like duplicate patient records and variations in data entry.
Integrating Issues
The main interoperability challenges encountered by healthcare data and analytics systems in connecting different platforms and legacy systems are the complexity that sometimes leads to data silos. In this case, isolated data repositories are created by various departments, which prevent comprehensive analysis and sharing of information.
Regulatory Compliance
Healthcare organizations need to handle complex regulatory requirements while implementing healthcare solutions based on data analysis. For example, access controls and data handling procedures require careful attention in HIPAA compliance.
Implementation Solutions
Best Practices
Healthcare data analytics platforms ensure that clear data governance policies and standardized procedures are in place. Organizations must ensure that such end-to-end solutions are secure enough to standardize data access and analysis safely while maintaining quality data.
Risk Mitigation Strategies
Moreover, healthcare organizations can also reduce their risks through the implementation of strong encryption, access controls, and conducting security audits frequently. They can automate management processes for data in order to reduce human error while facilitating the process.
Change Management
Improving data analysis healthcare should be approached as building up of a data-driven culture & collaboration with stakeholders. Processes with well-defined business needs and data that is available for quick instant effect should be emphasized.
Training Programs
Healthcare organizations should focus to develop an analytically capable workforce. To unfold the full potential of healthcare data analytics software, the staff requires skills in data analysis, statistics, and knowledge of platforms.
What is the role of data analytics in healthcare, you may ask; well it continues to evolve. It is in response to the challenges that solutions must be implemented at the organizational level. This means that three such areas can be better catered to by healthcare providers, so they can effectively invest in data analytics to produce improved patient care and operational efficiency.
Future Trends
Emerging Technologies
AI and Machine Learning Innovations
With the introduction of AI, healthcare data analytics platforms are transforming into something much more complex to analyze patient data. Advanced machine learning algorithms improve the accuracy of diagnosis, personalize treatment plans, and optimize clinical workflows. Healthcare data analytics software can now include predictive modeling that identifies potential health risks and recommends preventive measures.
Blockchain in Healthcare
Blockchain technology is changing healthcare data and analytics, providing safe and transparent sharing of data across healthcare networks. The innovation will facilitate patient-centric electronic health records, smart contracts for insurance processing, and increased supply chain transparency. Healthcare becomes more secure and efficient when data analytics is applied through the capability of blockchain to ensure data integrity but allow access to medical information.
Quantum Computing Potential
Healthcare analytics will change with quantum computing. It will revolutionize medical research, drug discovery, and personalized medicine through enhanced computation capabilities. Healthcare data analytics platforms that can leverage quantum computing are better at processing complex medical datasets, allowing for breakthroughs in genomics and treatment optimization.
Digital Twins and Real-time Analytics
Healthcare data analytics software now starts to increasingly use digital twin technology and creates virtual models of patients for predictive insights and personalized care. This advancement facilitates simulating treatment outcomes and real-time monitoring of patient health, signifying an important step forward in the conduct of data analysis healthcare.
AI-Based Diagnostic Tools
AI-driven diagnostic tools further add to the importance of data analytics in healthcare. The ability to analyze medical imaging at a much more accurate level, acceleration of disease identification, and providing possible treatment options on the basis of comprehensive patient data analysis are some of the examples.
Advances in big data analytics are making it possible for sophisticated analyses of health care with enhanced outcomes for patients. In many ways, this is transforming health care delivery into being more accurate, efficient, and more patient-centered yet retaining its strength in security and privacy standards.
Conclusion
Transformative changes in the healthcare environment have been brought about by data analytics, providing scope for better patient care and operational efficiency that had never been seen before. Healthcare data analytics is considered a tool of modern healthcare delivery without which no healthcare system can be visualized in the future. In this way, data analytics for healthcare is important in not only clinical applications but also for operational excellence and financial sustainability.
Data analytics in healthcare will be much more functional, including using AI, machine learning, and more advanced innovations, when healthcare organizations adopt all these technological developments. With better healthcare data analytics software, healthcare problems would be solved in more comprehensive ways. In the future, healthcare organizations should invest in robust analytics capabilities and follow a data-driven strategy to stay competitive and offer optimum care to the patient.